Note
Go to the end to download the full example code
Gradient of Radial kernels convolutions
This benchmark compares the performances of KeOps versus Numpy and Torch on various gradient of radial kernels convolutions. Namely it computes:
\[c_i^u = \sum_{j=1}^N \partial_{x_i^u} f\Big(\frac{\|x_i-y_j\|}{\sigma}\Big) \langle b_j, a_i \rangle, \quad \text{ for all } i=1,\cdots,M, \, u=1,\cdots,D\]
where \(f\) is a Gauss or Cauchy or Laplace or inverse multiquadric kernel. See e.g. wikipedia
Setup
Standard imports:
import numpy as np
import timeit
import matplotlib
from matplotlib import pyplot as plt
from pykeops.numpy.utils import grad_np_kernel, chain_rules
from pykeops.torch.utils import torch_kernel
from pykeops.torch import Vi, Vj, Pm
Benchmark specifications:
M = 10000 # Number of points x_i
N = 10000 # Number of points y_j
D = 3 # Dimension of the x_i's and y_j's
E = 3 # Dimension of the b_j's
REPEAT = 10 # Number of loops per test
use_numpy = True
use_vanilla = True
dtype = "float32"
Create some random input data:
a = np.random.rand(N, E).astype(dtype) # Gradient to backprop
x = np.random.rand(N, D).astype(dtype) # Target points
y = np.random.rand(M, D).astype(dtype) # Source points
b = np.random.rand(M, E).astype(dtype) # Source signals
sigma = np.array([0.4]).astype(dtype) # Kernel radius
And load it as PyTorch variables:
try:
import torch
use_cuda = torch.cuda.is_available()
device = "cuda" if use_cuda else "cpu"
torchtype = torch.float32 if dtype == "float32" else torch.float64
ac = torch.tensor(a, dtype=torchtype, device=device)
xc = torch.tensor(x, dtype=torchtype, device=device, requires_grad=True)
yc = torch.tensor(y, dtype=torchtype, device=device)
bc = torch.tensor(b, dtype=torchtype, device=device)
sigmac = torch.tensor(sigma, dtype=torchtype, device=device)
except:
pass
Convolution Gradient Benchmarks
We loop over four different kernels:
kernel_to_test = ["gaussian", "laplacian", "cauchy", "inverse_multiquadric"]
kernels = {
"gaussian": lambda xc, yc, sigmac: (
-Pm(1 / sigmac**2) * Vi(xc).sqdist(Vj(yc))
).exp(),
"laplacian": lambda xc, yc, sigmac: (
-(Pm(1 / sigmac**2) * Vi(xc).sqdist(Vj(yc))).sqrt()
).exp(),
"cauchy": lambda xc, yc, sigmac: (
1 + Pm(1 / sigmac**2) * Vi(xc).sqdist(Vj(yc))
).power(-1),
"inverse_multiquadric": lambda xc, yc, sigmac: (
1 + Pm(1 / sigmac**2) * Vi(xc).sqdist(Vj(yc))
)
.sqrt()
.power(-1),
}
With four backends: Numpy, vanilla PyTorch, Generic KeOps reductions and a specific, handmade legacy CUDA code for kernel convolution gradients:
speed_numpy = {i: np.nan for i in kernel_to_test}
speed_pykeops = {i: np.nan for i in kernel_to_test}
speed_pytorch = {i: np.nan for i in kernel_to_test}
print("Timings for {}x{} convolution gradients:".format(M, N))
for k in kernel_to_test:
print("kernel: " + k)
# Pure numpy
if use_numpy:
gnumpy = chain_rules(a, x, y, grad_np_kernel(x, y, sigma, kernel=k), b)
speed_numpy[k] = timeit.repeat(
"gnumpy = chain_rules(a, x, y, grad_np_kernel(x, y, sigma, kernel=k), b)",
globals=globals(),
repeat=3,
number=1,
)
print("Time for NumPy: {:.4f}s".format(np.median(speed_numpy[k])))
else:
gnumpy = torch.zeros_like(xc).data.cpu().numpy()
# Vanilla pytorch (with cuda if available, and cpu otherwise)
if use_vanilla:
try:
aKxy_b = torch.dot(
ac.view(-1), (torch_kernel(xc, yc, sigmac, kernel=k) @ bc).view(-1)
)
g3 = torch.autograd.grad(aKxy_b, xc, create_graph=False)[0].cpu()
torch.cuda.synchronize()
speed_pytorch[k] = np.array(
timeit.repeat(
setup="cost = torch.dot(ac.view(-1), (torch_kernel(xc, yc, sigmac, kernel=k) @ bc).view(-1))",
stmt="g3 = torch.autograd.grad(cost, xc, create_graph=False)[0] ; torch.cuda.synchronize()",
globals=globals(),
repeat=REPEAT,
number=1,
)
)
print(
"Time for PyTorch: {:.4f}s".format(
np.median(speed_pytorch[k])
),
end="",
)
print(
" (absolute error: ",
np.max(np.abs(g3.data.numpy() - gnumpy)),
")",
)
except:
print("Time for PyTorch: Not Done")
# Keops: generic tiled implementation (with cuda if available, and cpu otherwise)
try:
aKxy_b = torch.dot(ac.view(-1), (kernels[k](xc, yc, sigmac) @ bc).view(-1))
g3 = torch.autograd.grad(aKxy_b, xc, create_graph=False)[0].cpu()
torch.cuda.synchronize()
speed_pykeops[k] = np.array(
timeit.repeat(
setup="cost = torch.dot(ac.view(-1), (kernels[k](xc, yc, sigmac) @ bc).view(-1))",
stmt="g3 = torch.autograd.grad(cost, xc, create_graph=False)[0] ; torch.cuda.synchronize()",
globals=globals(),
repeat=REPEAT,
number=1,
)
)
print(
"Time for KeOps LazyTensors: {:.4f}s".format(
np.median(speed_pykeops[k])
),
end="",
)
print(
" (absolute error: ", np.max(np.abs(g3.data.numpy() - gnumpy)), ")"
)
except:
print("Time for KeOps LazyTensors: Not Done")
Timings for 10000x10000 convolution gradients:
kernel: gaussian
Time for NumPy: 1.7482s
Time for PyTorch: 0.0073s (absolute error: 0.029052734 )
Time for KeOps LazyTensors: 0.0014s (absolute error: 0.01171875 )
kernel: laplacian
Time for NumPy: 1.7831s
Time for PyTorch: 0.0088s (absolute error: 0.033813477 )
Time for KeOps LazyTensors: 0.0014s (absolute error: 0.037109375 )
kernel: cauchy
Time for NumPy: 1.6141s
Time for PyTorch: 0.0086s (absolute error: 0.021484375 )
Time for KeOps LazyTensors: 0.0015s (absolute error: 0.0126953125 )
kernel: inverse_multiquadric
Time for NumPy: 2.4614s
Time for PyTorch: 0.0077s (absolute error: 0.015625 )
Time for KeOps LazyTensors: 0.0017s (absolute error: 0.009765625 )
Display results
# plot violin plot
plt.violinplot(
list(speed_numpy.values()),
showmeans=False,
showmedians=True,
)
plt.violinplot(
list(speed_pytorch.values()),
showmeans=False,
showmedians=True,
)
plt.violinplot(
list(speed_pykeops.values()),
showmeans=False,
showmedians=True,
)
plt.xticks([1, 2, 3, 4], kernel_to_test)
plt.yscale("log")
# plt.ylim((0, .01))
plt.grid(True)
plt.xlabel("kernel type")
plt.ylabel("time in s.")
cmap = plt.get_cmap("tab10")
fake_handles = [matplotlib.patches.Patch(color=cmap(i)) for i in range(4)]
plt.legend(
fake_handles,
["NumPy", "PyTorch", "KeOps LazyTensors"],
loc="best",
)
plt.show()
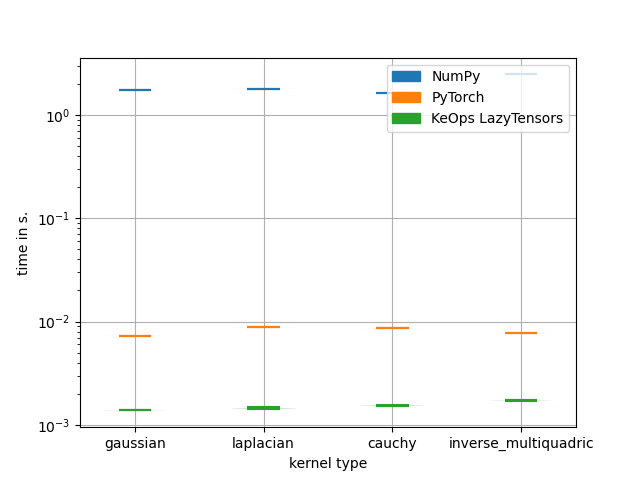
Total running time of the script: (0 minutes 31.101 seconds)