Note
Go to the end to download the full example code
K-NN on the MNIST dataset - PyTorch API
The argKmin(K)
reduction supported by KeOps pykeops.torch.LazyTensor
allows us
to perform bruteforce k-nearest neighbors search with four lines of code.
It can thus be used to implement a large-scale
K-NN classifier,
without memory overflows on the
full MNIST dataset.
Setup
Standard imports:
import time
import torch
from matplotlib import pyplot as plt
from pykeops.torch import LazyTensor
use_cuda = torch.cuda.is_available()
tensor = torch.cuda.FloatTensor if use_cuda else torch.FloatTensor
Load the MNIST dataset: 70,000 images of shape (28,28).
try:
from sklearn.datasets import fetch_openml
except ImportError:
raise ImportError("This tutorial requires Scikit Learn version >= 0.20.")
mnist = fetch_openml("mnist_784", cache=True, as_frame=False)
x = tensor(mnist.data.astype("float32"))
y = tensor(mnist.target.astype("int64"))
Split it into a train and test set:
D = x.shape[1]
Ntrain, Ntest = (60000, 10000) if use_cuda else (1000, 100)
x_train, y_train = x[:Ntrain, :].contiguous(), y[:Ntrain].contiguous()
x_test, y_test = (
x[Ntrain : Ntrain + Ntest, :].contiguous(),
y[Ntrain : Ntrain + Ntest].contiguous(),
)
K-Nearest Neighbors search
Perform the K-NN classification on 10,000 test images in dimension 784:
K = 3 # N.B.: K has very little impact on the running time
start = time.time() # Benchmark:
X_i = LazyTensor(x_test[:, None, :]) # (10000, 1, 784) test set
X_j = LazyTensor(x_train[None, :, :]) # (1, 60000, 784) train set
D_ij = ((X_i - X_j) ** 2).sum(
-1
) # (10000, 60000) symbolic matrix of squared L2 distances
ind_knn = D_ij.argKmin(K, dim=1) # Samples <-> Dataset, (N_test, K)
lab_knn = y_train[ind_knn] # (N_test, K) array of integers in [0,9]
y_knn, _ = lab_knn.mode() # Compute the most likely label
if use_cuda:
torch.cuda.synchronize()
end = time.time()
error = (y_knn != y_test).float().mean().item()
time = end - start
print(
"{}-NN on the full MNIST dataset: test error = {:.2f}% in {:.2f}s.".format(
K, error * 100, time
)
)
3-NN on the full MNIST dataset: test error = 2.95% in 0.49s.
Fancy display: looks good!
plt.figure(figsize=(12, 8))
for i in range(6):
ax = plt.subplot(2, 3, i + 1)
ax.imshow((255 - x_test[i]).view(28, 28).detach().cpu().numpy(), cmap="gray")
ax.set_title("label = {}".format(y_knn[i].int()))
plt.axis("off")
plt.show()
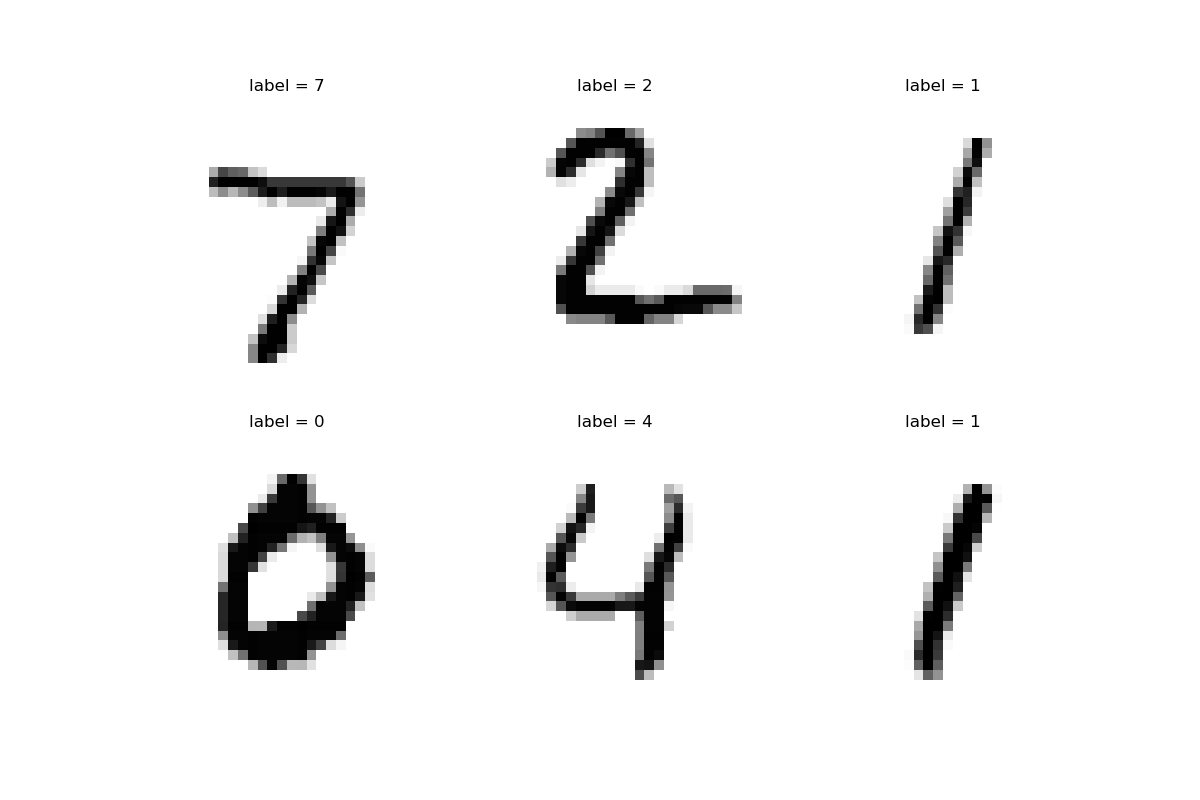
Total running time of the script: (0 minutes 3.797 seconds)